The Importance of Image Datasets for Classification in Modern Business
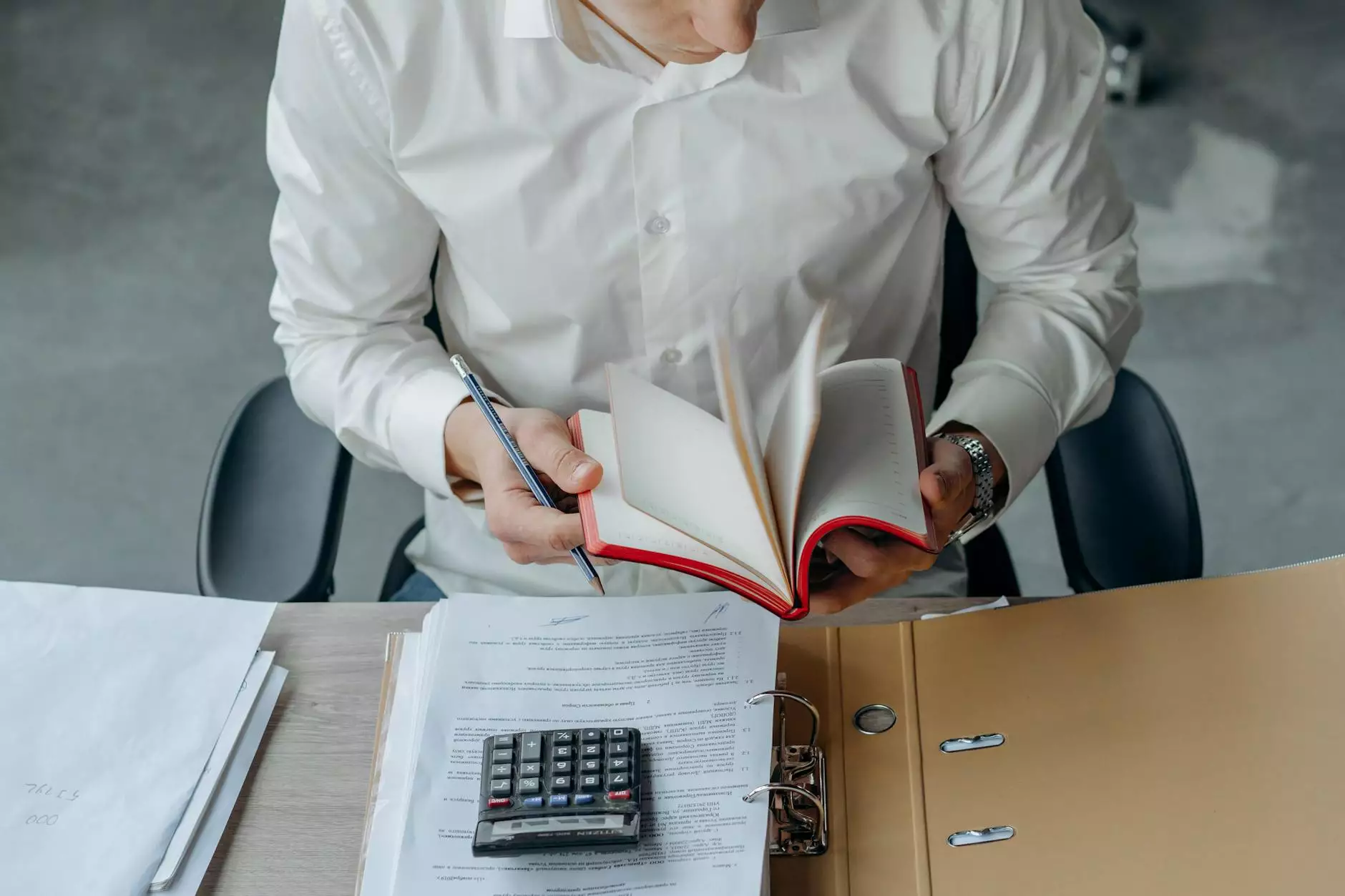
In an era where data reigns supreme, image datasets for classification have become a cornerstone for businesses looking to leverage cutting-edge machine learning technology. The ability to accurately classify images can bolster numerous applications, from facial recognition to object detection, ultimately improving efficiency and decision-making processes across various industries.
Understanding Image Datasets for Classification
At its core, image classification involves assigning a label to an image based on its visual content. This task is crucial in training machine learning models, which can recognize and categorize images autonomously. The models rely heavily on well-annotated image datasets, making data annotation tools essential for this purpose.
What are Image Datasets?
Image datasets are collections of labeled images that serve as a foundation for training machine learning models. They come in various shapes and sizes, with each dataset designed for specific classification tasks. The importance of using robust, well-structured datasets cannot be overstated, as the quality of these datasets directly impacts the performance of machine learning models.
The Role of Data Annotation in Creating Effective Image Datasets
Data annotation is the process of labeling data, in this case, images, to provide context that machine learning algorithms can understand. Effective data annotation involves:
- Precision: Accurate labeling ensures that the models learn from correct information.
- Consistency: Maintaining uniformity in labeling allows models to generalize better across different datasets.
- Scalability: Annotating large volumes of data efficiently is essential for training complex models.
Why Use Image Datasets for Classification?
Businesses today are leveraging image datasets for classification to enhance various operational aspects. Here are several reasons why these datasets are crucial:
1. Improved Automation
With accurately classified images, businesses can automate processes significantly. For example, in the retail sector, image classification can streamline inventory management by automatically identifying products from images, thus reducing manual input and errors.
2. Enhanced Customer Experience
In e-commerce, understanding customer preferences can be achieved through image classification. By analyzing user-uploaded images or photographs, businesses can curate personalized recommendations, thereby enhancing user experience and engagement.
3. Data-Driven Insights
Using image datasets for classification can yield valuable insights into consumer behavior. By employing machine learning techniques on classified images, businesses can uncover trends and patterns that inform product development and marketing strategies.
The Advantages of Using Keylabs.ai for Data Annotation
When it comes to creating high-quality image datasets, Keylabs.ai offers cutting-edge solutions that cater specifically to data annotation needs:
1. Advanced Annotation Tools
Keylabs.ai provides an array of data annotation tools designed to facilitate efficient and precise labeling. These tools include:
- Polygon and Bounding Box Annotation: Perfect for marking object outlines or specific areas of interest in images.
- Semantic Segmentation: Useful for identifying and classifying individual pixels within an image.
- Image Tagging and Categorization: Simplifies the process of labeling and organizing images based on predefined categories.
2. Intuitive Data Annotation Platform
The flexible and user-friendly interface of Keylabs.ai's data annotation platform ensures that teams, regardless of technical expertise, can annotate images effectively. This reduces the learning curve and increases productivity.
3. High Scalability
As businesses grow, so does the need for larger datasets. Keylabs.ai offers scalable solutions that can handle increasing volumes of images without sacrificing quality or speed.
Successful Applications of Image Classification in Business
Various industries have successfully implemented image classification technologies to optimize their operations. Below are some notable examples:
1. Healthcare
In healthcare, image classification plays a vital role in diagnostics. Machine learning models trained on annotated medical images can assist in identifying conditions such as tumors or fractures, thereby aiding medical professionals in providing timely treatment.
2. Automotive Industry
The automotive industry utilizes image classification for applications such as autonomous driving. By classifying road signs, obstacles, and lane markings, machines can make real-time decisions that ensure the safety of passengers.
3. Agriculture
Precision agriculture relies on image classification to monitor crop health. Drones equipped with cameras can capture images of farmlands, and with the help of annotated datasets, farmers can identify areas that require attention, ultimately optimizing yields.
Challenges and Considerations in Image Classification
While the potential of image datasets for classification is immense, several challenges must be overcome to maximize their effectiveness:
1. Ensuring Data Quality
The success of machine learning models heavily depends on the datasets used for training. Therefore, ensuring data quality through rigorous annotation processes is paramount. Inconsistent labeling or poor-quality images can lead to ineffective models.
2. Addressing Bias in Datasets
Bias in training datasets can lead to skewed results, which can adversely affect the performance of models in real-world applications. It is essential to curate diverse datasets that represent a wide range of scenarios to mitigate this issue.
3. Scaling Annotation Efforts
As demand for more extensive datasets grows, businesses must find efficient ways to manage and scale annotation efforts. Solutions like keylabs.ai's annotation platform help address this issue by automating and simplifying the annotation process.
Conclusion: Unleashing the Potential of Image Datasets for Classification
In conclusion, image datasets for classification are transforming how businesses operate by providing the means to automate processes, enhance customer experiences, and drive data-driven decision-making. With tools and platforms like Keylabs.ai, businesses can create high-quality, well-annotated datasets that significantly improve the performance of machine learning models.
As the landscape of machine learning continues to evolve, the emphasis on effective data annotation and robust dataset management will undoubtedly shape the future of various industries. Companies that prioritize these aspects will not only gain a competitive edge but will also be at the forefront of innovation in their respective fields.