Unlocking the Power of Annotation in Machine Learning for Business
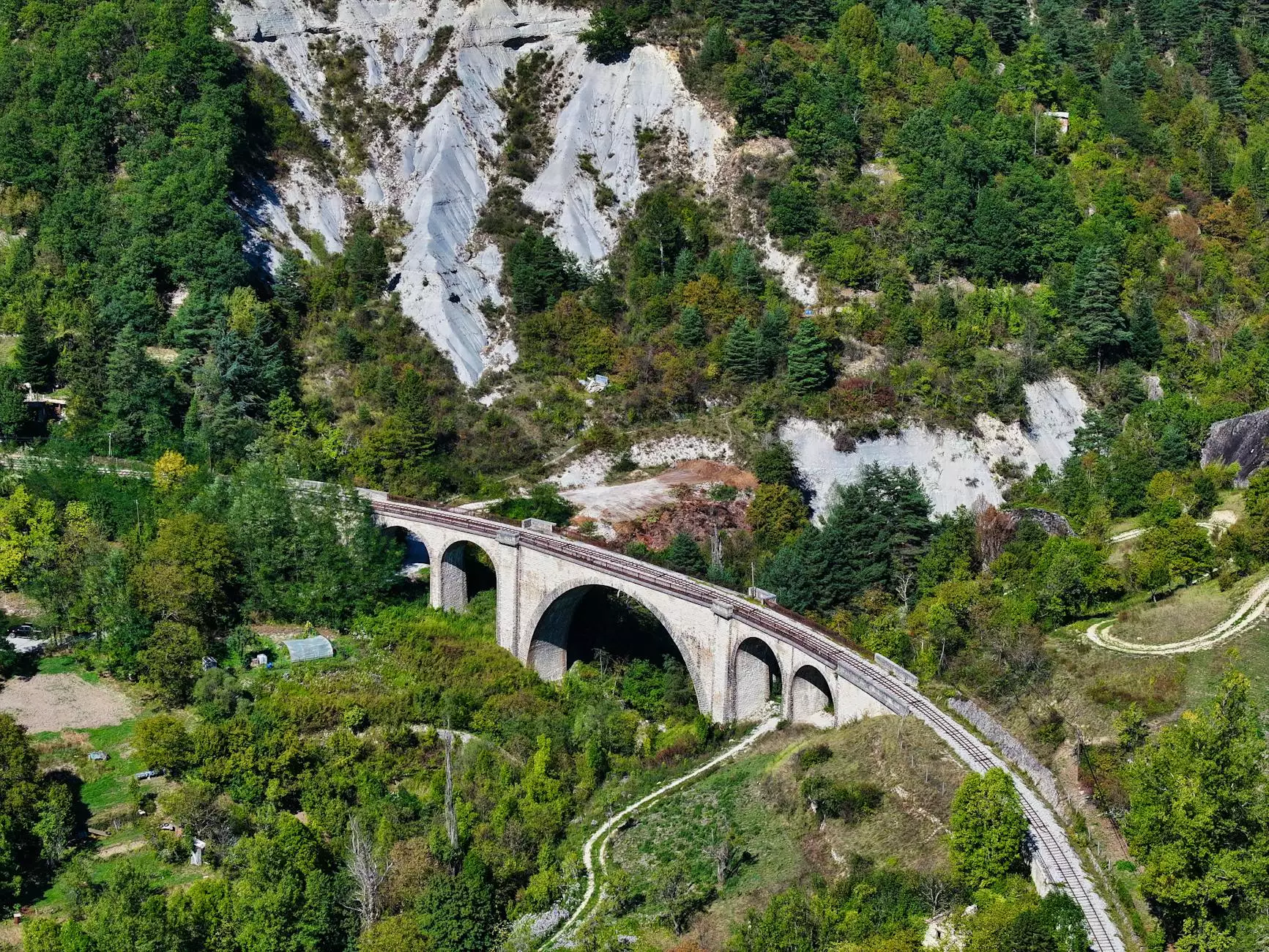
In today's digitally driven world, the fusion of technology and business has created unprecedented opportunities. Annotation in machine learning is a pivotal aspect that organizations must embrace to optimize operations, particularly in service-oriented sectors such as Home Services and Keys & Locksmiths. This article delves into the transformative role that data annotation plays in machine learning and how businesses can leverage it effectively for growth and efficiency.
What is Annotation in Machine Learning?
Annotation in machine learning refers to the process of labeling data to train machine learning models. This step is crucial because the quality and accuracy of the data annotations directly impact the performance of the algorithms. By providing context to raw data, annotations enable machines to learn patterns, make predictions, and derive insights from previously unseen data.
Types of Annotations
There are various types of annotations utilized across different domains:
- Image Annotation: Involves labeling images to identify objects, features, or actions within them, which is essential for applications in computer vision.
- Text Annotation: Involves marking up text documents to highlight specific entities, relationships, or sentiments, often used in natural language processing (NLP).
- Audio Annotation: Involves labeling audio clips, which is vital for developing voice recognition systems.
- Video Annotation: Involves tagging frames in videos to detect and categorize actions, significant in surveillance and driver assistance systems.
The Importance of Annotation in Machine Learning for Businesses
In the realms of home services and locksmith industries, leveraging annotation in machine learning can lead to improved customer experiences, operational efficiencies, and enhanced security measures. Here's how:
1. Enhancing Customer Service
Utilizing machine learning models trained on annotated data allows businesses to analyze customer interactions efficiently. By deriving insights from customer behavior, organizations can tailor services and provide instantaneous support. For instance, a locksmith business can implement an AI-driven chatbot that understands customer inquiries through text annotation, offering relevant responses and solutions based on past interactions.
2. Streamlining Operations
Machine learning models can optimize logistics and operational processes. For instance, consider a home service company that conducts regular maintenance schedules. By annotating past service records, machine learning algorithms can predict when a customer is likely to need servicing again, ensuring timely follow-ups and reducing downtime.
3. Enhancing Security Systems
For locksmiths and security service providers, the use of video annotation technologies enables the creation of sophisticated surveillance systems. These systems can accurately identify unusual activities by analyzing annotated surveillance footage, thereby preventing unauthorized access and increasing overall security for clients.
Implementing Annotation in Machine Learning: A Step-by-Step Guide
To effectively utilize annotation in machine learning, businesses need a structured approach. Here’s a step-by-step guide:
Step 1: Identify the Data Type
Begin by determining what type of data is essential for your business operations – be it text, images, audio, or video. Understanding your needs will dictate the type of annotation required.
Step 2: Choose Annotation Tools
Select appropriate annotation tools that suit your specific needs. For instance, tools that support image or video annotation should be user-friendly and capable of handling the volume of data involved.
Step 3: Train Your Annotation Team
Even with the best tools, accurate annotations rely heavily on the skill of the annotators. Invest in training your team to ensure they understand the context and relevance of the annotations required.
Step 4: Implement Quality Control
Set up quality control measures to ensure that annotations are accurate and consistent. Regular audits and feedback can help maintain high standards.
Step 5: Integrate and Test Your Model
Once your data is annotated, integrate it into your machine learning model. It’s crucial to test the model thoroughly to ensure that it performs well with the annotated data.
Case Studies Highlighting the Benefits of Annotation in Machine Learning
Case Study 1: Locksmith Business Optimization
A locksmith service implemented a machine learning system that utilized annotation in machine learning to analyze previous lockout scenarios. By labeling data about customer locations, lock types, and time of service, they developed predictive models that improved dispatching efficiency, reduced waiting time, and increased customer satisfaction. They reported a 30% increase in repeat clients due to enhanced service quality.
Case Study 2: Home Service Enhancement
In a home service scenario, a company employed annotated audio data from customer calls to train a natural language processing system. This system could effectively route calls to the right service specialists based on customer needs. As a result, they observed a 25% decrease in call resolution time, directly impacting their operational efficiency.
Challenges in Annotation for Machine Learning
While the advantages of annotation in machine learning are substantial, several challenges exist:
1. Scalability
As data volume grows, keeping up with annotation can become overwhelming. Systematic processes and possibly automated tools can help alleviate this burden.
2. Skill Gaps
Finding skilled annotators who understand the nuances of the domain is crucial for high-quality results. Ongoing training and clear guidelines can bridge this gap.
3. Quality Assurance
Maintaining consistency and accuracy in annotations is vital. Regular reviews and the use of multiple annotators for the same datasets can help ensure quality.
Conclusion: The Future of Annotation in Business
In conclusion, annotation in machine learning is not just a technical requirement; it is a pivotal strategy that can transform how businesses in home services and keys & locksmiths function. By effectively leveraging this technology, organizations can enhance customer service, streamline operations, and improve security measures. As the technology continues to evolve, staying abreast of developments in data annotation will be crucial for businesses seeking competitive advantages in the digital marketplace.
Embracing the future of annotation in machine learning is not just about keeping up; it’s about leading the industry and setting new benchmarks for excellence.